Optimization of the irrigation schedule for a given crop at a specific location
Model-Based Optimization of Irrigation Scheduling
Raphael Linker, linkerr@technion.ac.il
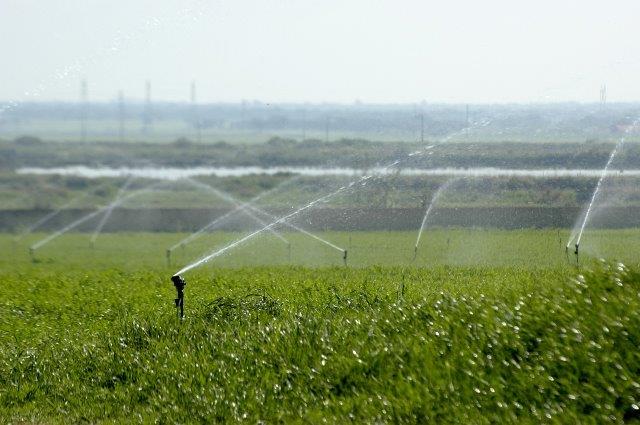
modern intensive agriculture relies on supplemental irrigation in order to achieve high yields. however, diminishing water resources and increasing demand from urban and industrial sectors are restricting the amount of water available to agriculture.
in order to ensure agriculture sustainability, it is imperative to ensure that irrigation water is applied appropriately and water wasting is minimized.
improving water use efficiency is a multi-level problem that includes, among others, choice of the crop(s), crop variety, irrigation method and irrigation timing. our work focuses on the latter, namely optimization of the irrigation schedule for a given crop at a specific location.
although it is well established that timely irrigation can increase substantially irrigation efficiency and water productivity, determining the adequate timing and amount of irrigation remains a very challenging task for farmers. numerous studies have considered the development of decision support systems (dss) which would help farmers manage irrigation more efficiently.
such systems can be broadly divided into two categories: rule-based and model-based. the main difference between these two approaches is that in rule-based approaches knowledge about the crop-soil-atmosphere interaction is included implicitly in the decision rules themselves while in model-based approaches there is a clear distinction between the model, which describes the crop-soil-atmosphere interaction, and the decision stage that makes use of that model.
in our work we adopt the model-based approach, which has the main advantage that it is possible to develop the decision stage in such a way that its use is not restricted to a specific model (or even crop) but rather models can be swapped in an almost plug-and-play fashion.
in other words, this approach allows to take advantage of crop-soil-atmosphere models available in the literature (e.g. aquacrop, ceres, stics, epic) and to focus on the development of the decision-making stage, for which we adopt a dynamic optimization framework.
according to this framework, the crop-soil-atmosphere model is used to predict the crop (and soil) response to a wide range of possible decisions (i.e. irrigation schedule) in order to determine the best set (s) of decisions.
such an approach has two main drawbacks: (1) the crop-soil-atmosphere model is only an imperfect description of the actual system, and (2) predicting crop development requires assumptions about the weather during the period over which the optimization is performed (so-called optimization horizon).
1) the crop-soil-atmosphere model is only an imperfect description of the actual system
our current research focuses on quantifying the impact of model and forecasts imperfectness on the overall performanceof a model-based decision support system based on dynamic optimization framework, and developing new procedures that reduce the impact of these imperfections.
2) predicting crop development requires assumptions about the weather during the period over which the optimization is performed
with respect to weather forecasts uncertainty, we are developing deterministic and stochastic approaches and comparing their respective performances. in deterministic approaches perfect forecasting of the future weather is assumed, which is of course unrealistic but has the advantage of greatly simplifying the optimization problem.
although such deterministic approaches may seem overly simplistic to be of practical use, a number of simulation studies we conducted with actual forecasts at several locations throughout europe and the us showed that by recomputing the optimal irrigation schedule frequently (every few days) using updated information about the past weather and updated forecasts, it is possible to achieve results which are very close to optimal in terms of water use efficiency (wue).
whereas deterministic approaches unrealistically assume perfect knowledge of future weather, stochastic approaches consider a number of possible forecasts (so-called forecast ensemble), each one with some probability of occurrence. as a result, uncertainty about the future weather is taken into account explicitly during the decision-making process. however, compared to deterministic approaches, stochastics approaches are much more complex to develop and much more demanding computation-wise.
one of our current focuses is systematic comparison between these different approaches in order to quantify the advantages of specific procedures, given the degree of accuracy/reliability of current weather forecasts.
with respect to imperfectness of the crop-soil-atmosphere models, most commercial farmers perform some kind of measurement of the crop and/or soil status during the season and we are developing new methodologies to correct the model in real-time as these measurements become available. a number of factors make such data assimilation very challenging: the reliability and accuracy of measurements performed in-situ tend to be low; the number of measurements is typically small, both in terms of spatial and temporal resolutions; some of the measurements do not have a straightforward equivalent in the crop-soil-atmosphere models.we are currently developing several approaches that address these issues.