The Importance of Agri-data in Weed Management
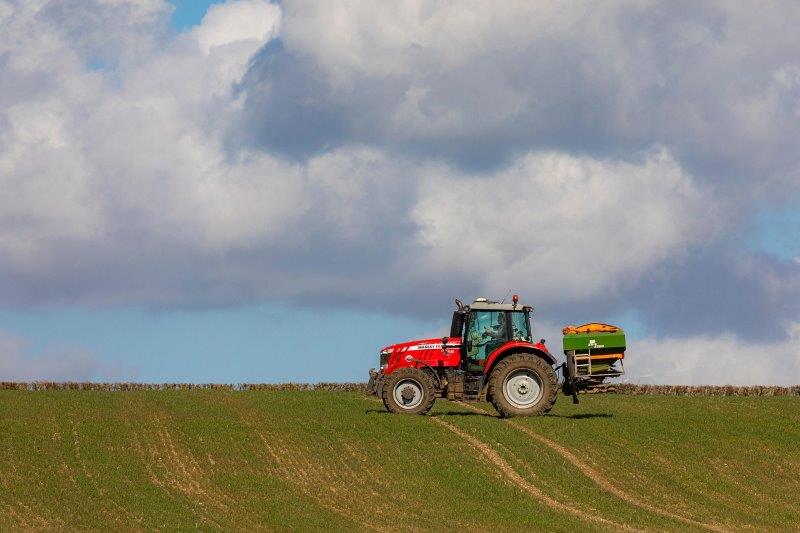
Roni Gafni roni.ga@migal.org.il *
When modeling an agroecosystem, our goal may be to describe a phenomenon, quantify the influence of various factors, or predict outcomes under certain conditions. This could involve analyzing the spatio-temporal distribution of diseases, pests, or weeds in a field or using machine learning and other AI tools to predict optimal timing for management actions. Another approach is to apply statistical multivariate models that attempt to explain the factors influencing a system despite considerable variability or “noise”. This method is commonly applied in ecological research. However, agricultural systems differ significantly from natural systems. They are highly disturbed environments with substantial heterogeneity both between and within fields.
This variability stems from factors such as field location, climate conditions, pest pressures, and management strategies. Perhaps the most influencing factor is the human element, as each farmer makes unique management decisions. Adding further complexity, one must also consider the interactions between all these factors. Managing such variability effectively requires a broad representation of diverse fields across regions and over multiple years or growing seasons. This approach enables the development of multivariate models capable of filtering through the noise and identifying the most relevant factors. However, it is seldom used due to its time-consuming and complex nature. The main challenge lies in the significant effort required to collect the data, whether through scouting numerous commercial fields during a short period or over several years, or by gathering management data directly from individual farmers in the absence of an accessible database. Despite these challenges, the potential of such models to enhance our understanding of how management practices and other variables affect the agroecosystem is vast, making the effort worthwhile.
In agricultural data, or Agri-data, the chronology of all activities performed by a grower are captured, as well as every input invested in a field throughout the growing season. This includes tasks such as soil preparation before and during planting, pest control applications, irrigation, fertilization, and more. Additionally, external factors such as climate conditions, soil type, altitude, slope, and the surrounding environment—whether natural, urban or agricultural— may influence crop development, yield and post-harvest parameters. This valuable information, recorded by farmers over many years, plays a critical role in optimizing agricultural practices. The data is primarily collected to meet the demands of regulatory bodies, which farmers— especially those exporting their produce—must comply with. However, in recent years, there has been growing interest from other parties, such as companies offering agricultural data collection and management services aimed at providing data-driven analyses, as well as agricultural research and extension services seeking to leverage Agri-data to explain large-scale phenomena and develop region-wide recommendations.
In the field of weed science, there is growing recognition that understanding the dynamics of weed populations in agricultural fields requires integrating both spatial variability across multiple fields and temporal variability among and within growing seasons. While many studies examine weed communities in arable fields, there are considerably fewer studies that investigate comprehensively the factors influencing weed disturbances, supported by extensive sampling and corresponding management data.
To highlight the potential of this modeling approach, I will review two studies on weed infestation conducted in commercial processing tomato fields across four growing regions in northern Israel. This geographic region is characterized by a unique climate gradient ranging from semi-arid conditions in the eastern Beit She’an Valley to Mediterranean conditions in the western Zevulun Valley and northern Hula Valley. The significant difference in climate conditions among growing regions affect management practices such as planting season and timing of application, in addition to biotic factors such as growth rate.
The first study by Cohen et al. (2017), investigated the factors influencing broomrape (Phelipanche aegyptiaca) infestations in commercial tomato fields. This research involved extensive sampling and data collection on both current and historical levels of broomrape infestation across various growing regions, examining factors such as crop rotation, historical infestation levels, and proximity to infected fields. By intensively mapping 43 fields, the study provided a comprehensive within-field characterization. The findings highlighted the westward spread of broomrape from the Beit She’an Valley to the Hula Valley (Fig. 1) and underscored the role of tomato as a host crop in exacerbating infestations, both within fields and in neighboring fields.
Figure 1. Mean infestation levels of P. aegyptiaca across processing tomato growing regions in northern Israel at different time periods, taken from Cohen et al., 2017.
The second study, which includes my own doctoral research under the supervision of Prof. Hanan Eizenberg and Dr. Lior Blank, from the Agricultural Research Organization at Newe Ya’ar and Volcani center, focused on Amaranthus infestations in commercial tomato fields. Over two consecutive growing seasons, we mapped close to 130 fields across northern Israel and collected management data for 103 of them (Fig. 2). Our statistical models identified factors such as herbicide application frequency and rainfall occurrence following weed treatment as contributors to Amaranthus infestation levels (Fig. 3). Insights gained from this modeling process laid the foundation for field experiments conducted over two growing seasons across different growing regions. These experiments tested variables such as application timing, intensity, and integrated management approaches (Fig. 4), ultimately providing region-specific management insights.
Figure 2. Map of Israel (A), with a gray square designating the study region. Mean maximum temperatures during the month of March (B) and mean annual precipitation (C) for the period of 1983–2017 display the climate gradient in Northern Israel. The four major growing areas of processing tomatoes in the northern region– Beit She’an Valley (BS), Jezreel Valley (Jz), Zevulun Valley (Zv) and Hula Valley (Hul) – are indicated by gray polygons showing the 58 and 45 fields (black polygons) surveyed during the 2018 (D) and 2019 (E) growing seasons, respectively. From Gafni et al., 2023.
Figure 3. Tables 4 and 5 reproduced from Gafni et al., (2023),showing parameter estimates for the selected models for moderate and severe Amaranthus infestation rates, respectively. Management parameters are highlighted in purple and climate parameters in blue (added for emphasis). The pre-planting application of sulfosulfuron, an acetolactate synthase (ALS) inhibitor herbicide, is applied to control broomrape infestation. Although it was not directly applied to target Amaranthus species, this management variable was included in the model due to the documented effect of pre-emergence ALS-inhibitors application on Amaranthus infestation.
In conclusion, the growing recognition of the importance of broad-scale data collection in addressing weed management and other agronomic questions underscores the value of Agri-data. Research such as this addresses regional-scale questions while considering spatial variability both within and between fields, offering a perspective that enables location-targeted management under varying climate conditions. I encourage farmers and other relevant entities to share information in order to create a robust database capable of supporting complex analyses, thereby advancing knowledge and providing collective benefits. Models are not the end goal; they are steps along the way. By harnessing Agri-data, we can build more effective models and improve weed management strategies.
Figure 4. Field experiment conducted in 2021 at Eden Farm, Beit-She’an Valley, assessing the effect of intensity and timing of application on Amaranthus infestation in commercial processing tomato fields. This experiment was one of nine field trials based on modeling results and included the integration of finger-weeder cultivation to reduce chemical spraying.
*Cohen, Y., Roei, I., Blank, L., Goldshtein, E., Eizenberg, H., 2017. Spatial spread of the root parasitic weed Phelipancheaegyptiaca in processing tomatoes by using ecoinformatics and spatial analysis. Front. Plant Sci. 8, 973. https://doi.org/10.3389/fpls.2017.00973
*Gafni, R., Ziv, G.A., Eizenberg, H., Blank, L., 2023. A regional-scale study of the contribution of local, management and climate factors to the infestation of processing tomato fields with Amaranthus species. Eur. J. Agron. 143, 126722 https://doi.org/10.1016/j.eja.2022.126722
*Northern R&D (Mop Tzafon), MIGAL, Kiryat Shemona, Israel.