Plant protection -‘red lines’ and AI
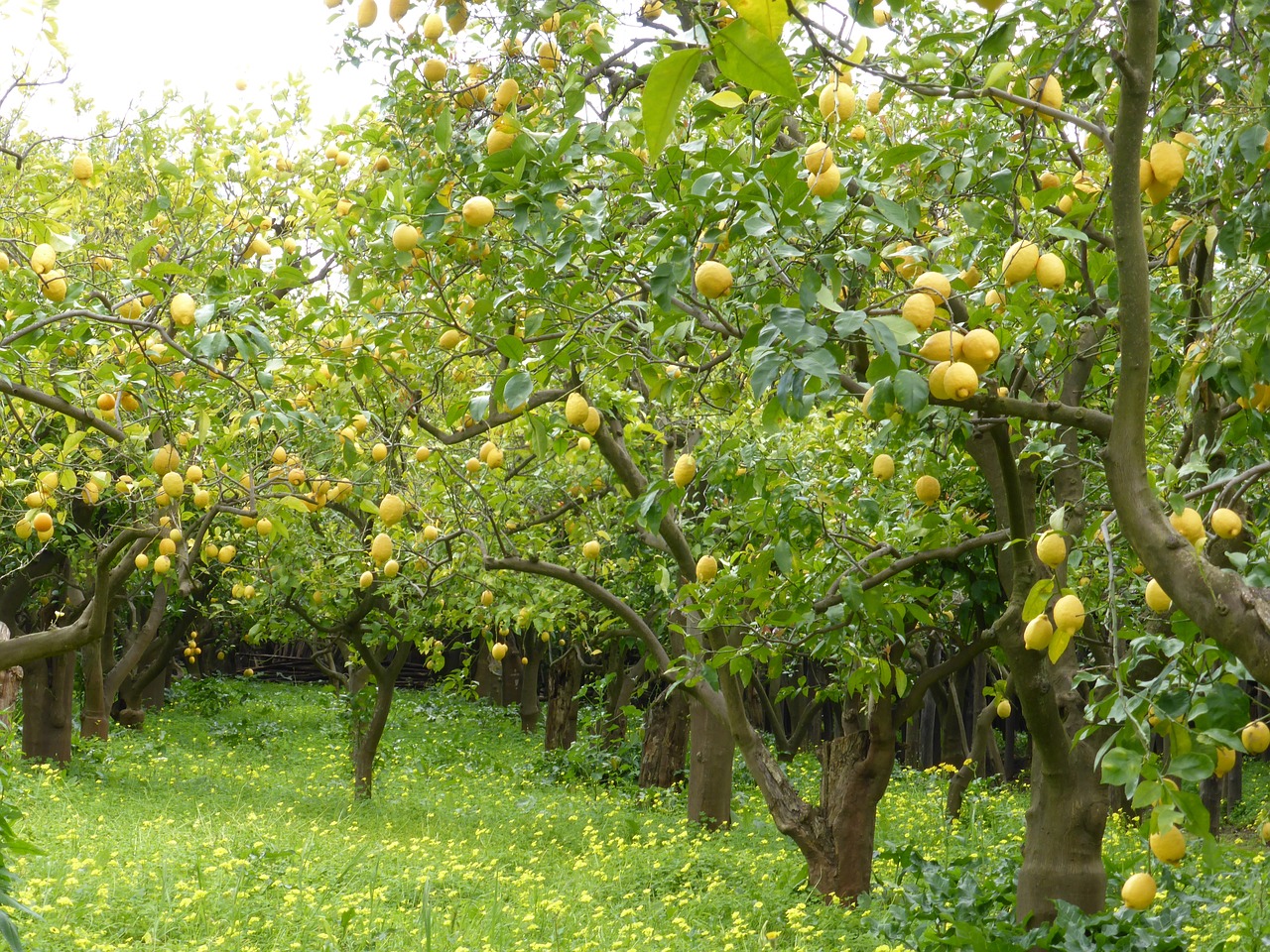
Chen Keasar(1), Tamar Keasar(2), and Saskya van Nouhuys(3)
Crop pests – weeds, pathogens, and insects – regularly challenge farmers and extension specialists. Chemical pesticides are the main means of pest control in conventional agriculture, but many products have been banned over the last decades. Other pesticides are declining in efficiency as pests become resistant to them. These constraints, combined with concerns for human and environmental health, drive the Integrated Pest Management (IPM) approach to reduce pesticide use. Farmers tend to avoid risks when dealing with crop pests. They spray their fields once pests are spotted (or even earlier, when pests are expected to arrive) to avoid yield losses. IPM, on the other hand, advocates spraying exactly as needed. Too little spraying might reduce crop yields, while too much spraying increases the farmers’ expenses, health risks, and marketing challenges.
The ‘red lines’ – action thresholds: In this article we focus on action thresholds, key decision support tools in IPM. A field is sprayed only when its pest populations exceed a pre-decided static threshold. In other words, the action threshold is a red line that calls for action when crossed. Below the threshold, the pest’s expected damage to crop yield is lower than the cost of control, and spraying is economically unjustified. Above the threshold, spraying makes economic sense and is recommended. Accordingly, the threshold calculation considers the pest-induced yield loss, the crop’s market price, and the pesticides’ cost and efficiency. Action threshold values vary among different crops and pests. The main challenge in this approach is tracking the pests in the field and predicting their rates of increase and crop damage. Farmers fear irreversible crop damage if they fail to identify that an action threshold had been crossed. We aim to combine biological information with computational methods to help identify the pests and to estimate their population growth accurately.
Natural enemies of crop pests are predators and parasites that help protect crop plants by reducing pest populations. When natural enemies are sufficiently common, insecticide use can be reduced. On the other hand, insecticides harm natural enemies through direct toxicity and by reducing their prey. This calls for adjustment of the action thresholds to the density of the natural enemies in the field: raising the threshold (spray less) when natural enemies are abundant and lowering the threshold (spray more) when they are scarce. Such adjustments are called ‘dynamic action thresholds’. Using a theoretical model, we found that farmers can reduce insecticide use, with no profit loss, by using dynamic action thresholds. The predicted benefits of using dynamic thresholds increase for mobile insects and when pesticide efficiency is low.
The barriers: Despite their advantages, dynamic thresholds are not yet in wide commercial use. They have been applied in some countries to control moth pests in tomatoes, thrips in strawberries, and aphids in cotton, soybean, and wheat. Nevertheless, most pest control programs worldwide either rely on static thresholds or do not use action thresholds at all. To apply dynamic thresholds, both pests and natural enemies must be monitored in the fields, and monitoring is limited by the availability, time, and expertise of pest inspectors. User-friendly, cheap and reliable monitoring tools are needed to overcome this considerable barrier, and to support informed pest control decisions.
AI for insect monitoring: Insect monitoring increasingly relies on AI-supported systems, which include hardware devices to collect insect images and software to identify specific species. The software is ‘trained’ on many examples of the target insects, and its performance improves as the set of training images grows. Most existing systems track crop pest such as moths, flies and aphids. By automatically identifying and counting the pests, such systems can help implement static action thresholds. The same software can also be trained to identify the pests’ natural enemies in the set of insect images, with no need for additional sampling in the field. This paves the way for wider use of dynamic thresholds in IPM.
A case study – action thresholds for the Asian citrus psyllid in India: Our ongoing research illustrates how automated insect monitoring can aid pest control. The Asian citrus psyllid (Fig. 1) is an insect that feeds on citrus trees and on related plants. The psyllid’s feeding only mildly damages the plants, but it is nevertheless considered a serious pest because it transmits the greening disease, which is lethal to citrus. The disease has no effective treatment, therefore control efforts focus on the psyllids. Insecticides are the main control method in India but are costly and not available to all farmers. Consequently, many citrus orchards receive no treatment against the psyllid.
Fig. 1: The Asian citrus psyllid on a yellow sticky trap
The psyllid originates from the far east but has spread to America (infesting large citrus areas in Florida and California) and has recently invaded Israel as well. The psyllid’s main natural enemy in Eastern Asia is the parasitic wasp Tamarixia radiata. This wasp was imported into the USA, where it successfully controls the pest in some areas. We are developing protocols to monitor the psyllid and its natural enemy, to allow management of this pest using dynamic action thresholds. We are using STARdbi, our AI-assisted online system, to store, catalog, and identify insect images. Fig. 2 describes the workflow of our project.
STARdbi has some unique features that facilitate the task. First, it uses low-cost and accessible sticky cards for insect trapping, and this enables us to sample widely within and across fields. Second, we can adapt the software to identify all kinds of flying insects. Therefore, we can use it for diverse purposes, such as tracking of medical pests or estimating insect biodiversity. Finally, the image database is free, shareable, and can be queried for multiple aims. For example, trap images that were originally placed to detect a specific pest can also inform on the total abundance of insects and on their body sizes.
To sum up: We hope that AI-assisted monitoring of insects will enable widespread use of dynamic thresholds in IPM. We welcome collaborations with farmers, extension specialists, and crop protection researchers in applying STARdbi towards healthier and more sustainable agriculture.
Acknowledgements: Our project is supported by an international research grant, funded by the Israeli Ministry of Science and the Indian Department of Science and Technology.
Figure 2- Schematic workflow in the STARdbi system
(1) Department of Computer Science, Ben Gurion University, Israel
(2) Department of Biology and the Environment, University of Haifa, Israel, email: tkeasar@research.haifa.ac.il
(3) Centre for Ecological Sciences, Indian Institute of Science, India